Artificial Intelligence and Racial Justice in the Criminal System
- Human Rights Research Center
- Nov 28, 2023
- 2 min read
Author: Serena Malik
November 28, 2023
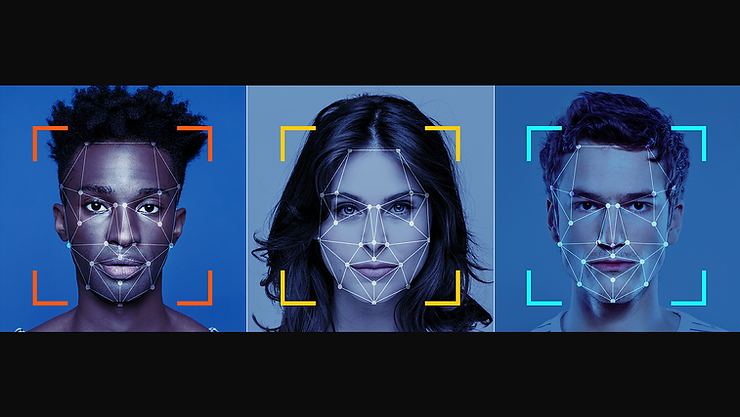
In today's rapidly advancing digital age, Artificial Intelligence (AI) is often hailed as a beacon of efficiency and objectivity, particularly within the criminal justice system. However, beneath its potential lies a significant concern: perpetuating, and at times amplifying, racial biases entrenched in historical data. As we journey through the transformative potentials and pitfalls of AI in criminal justice, a pressing question emerges: How can we ensure racial justice in AI applications?
The criminal justice system, like many societal institutions, has been plagued by systemic racial disparities. For instance, a study conducted by the National Association for the Advancement of Colored People (NAACP) found that African Americans are incarcerated at more than five times the rate of white Americans.
When AI systems are trained on historical data from such a system, they can inadvertently encode these biases. A landmark investigation by ProPublica in 2016 revealed that an AI system used to predict future criminal behavior was biased against Black defendants. This software, meant to guide sentencing, perpetuated racial disparities.
On one hand, AI tools, such as facial recognition, can assist law enforcement in swiftly identifying suspects and solving crimes. Yet, studies like the one conducted by the MIT Media Lab indicate racial and gender biases in facial recognition systems, which often misidentify women and people of color. Such misidentifications can lead to wrongful arrests, eroding the trust of communities in the very technologies meant to protect them.
Achieving racial justice in AI requires a multi-pronged approach:
Bias Detection & Correction: Before deploying any AI system in the justice system, rigorous tests for racial and ethnic biases should be conducted. This can be informed by works like Buolamwini's and Gebru's research, which provides methodologies for bias detection.
Transparency & Accountability: AI's decision-making processes, especially in the justice domain, should be transparent. As recommended by the Partnership on AI, systems should be designed in a way that their predictions can be interpreted and questioned.
Diverse Training Data: To reduce racial bias, AI systems should be trained on diverse datasets. Organizations like AI Now Institute emphasize the importance of diverse training data to combat racial biases.
Community Engagement: Engaging communities, especially those most affected by AI's decision-making in criminal justice, ensures that technology aligns with the values and needs of the people it serves.
While AI holds immense potential to streamline and augment the capabilities of the criminal justice system, it remains crucial to address its inherent biases. Only by actively working to understand and mitigate these biases can we hope to realize the promise of AI in fostering a just and equitable society.